With the proliferation of artificial intelligence and the next-generation communication technology, the growing demand for high-performance computing has driven the development of custom hardware to accelerate this specific category of computing. However, processors based on electronic hardware have hit the bottleneck of unsustainable performance growth as the exponential scaling of electronic transistors reaches the physical limit revealed by Moore’s law. In emerging artificial intelligence applications, massive matrix operations require high computing speed and energy efficiency. Optical computing can realize high-speed parallel information processing with ultra-low energy consumption on photonic integrated platforms or in free space, which can well meet these domain-specific demands. Optical computing compute with photons instead of electrons, and therefore optical computing can dramatically accelerate computing speed by overcoming the inherent limitations of electronics.

Figure 1. Principles and applications of mainstream architectures for photonic matrix computing.
To some extent, neuromorphic engineering is an attempt to move computational processes of artificial intelligence algorithms to specific hardware, enabling functions that are difficult to realize with conventional computing hardware. In complementary metal–oxide–semiconductor (CMOS), matrix operations are typically implemented by systolic arrays or single instruction multiple data (SIMD) units. Due to the nature of electronic components, performing simple matrix operations requires a large number of transistors working together and additional schedulers to coordinate data movements involving weights. In optical computing, matrix computing can be easily implemented by passing through basic photonic devices, such as Microring resonator (MRR), Mach-Zehnder interferometer (MZI) and diffractive planes, as well as their composition array or system. Therefore, from the perspective of neuromorphic engineering, optical computing is more consistent with the mathematical nature of matrix computing than electronic computing. Unlike electrical circuit technologies, photonic circuits have some extraordinary properties such as ultra-wide bandwidth, low latency, and low energy consumption. Furthermore, light has several dimensions such as wavelength, polarization, and spatial mode to enable parallel data processing, resulting in remarkable acceleration against a conventional von Neumann computer, which makes the optical computing approach a viable and competitive candidate for artificial intelligence accelerators.
This paper introduces the basic principles of three different optical computing architectures based on multiple plane light conversion (MPLC), on-chip MRR arrays, and on-chip MZI mesh, and also expounds the photonic matrix computing based on different optical computing architectures as well as the latest research progress in photonic artificial intelligence hardware. The performance parameters of representative optical computing architectures in recent years are summarized, the current challenges and development trends of optical computing are analyzed, and the prospects for further improvement of optical computing architectures are put forward.
Unlike integrated schemes such as microring and MZI matrix cores, the MPLC matrix core builds computing capabilities directly above an optical field propagating in free space. Among these three methods, MPLC was the first to be implemented in optical computing, and the initially programmable matrix–vector multiplication (MVM) was finished with spatial optical elements. The MPLC matrix core is the only one that can currently support super-large-scale matrix operation, which makes it valuable in pulse shaping, mode processing, and machine learning.
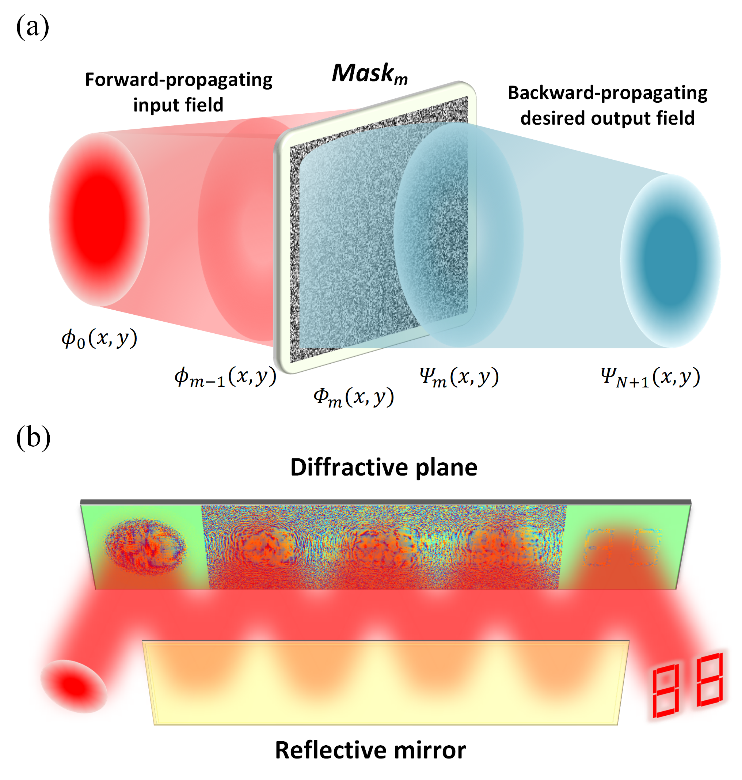
Figure 2. The principle of the MPLC matrix core. (a) schematic diagram of the wavefront matching method based on one diffractive plane. (b) the MPLC matrix core is composed of a series of diffractive planes encoded with amplitude and phase information.
The microring has a very compact structure and its radius can be as small as a few microns, which means the footprint of photonic devices can be greatly reduced, and thus the integration density can be competitive. The microring has been widely used in on-chip WDM systems, filtering systems, etc. In addition, a microring array can also be used in the operations of incoherent matrix computation since each microring can independently configure the transmission coefficient of a wavelength channel. Therefore, the microring matrix core is well suited to implement WDM-MVM operation.
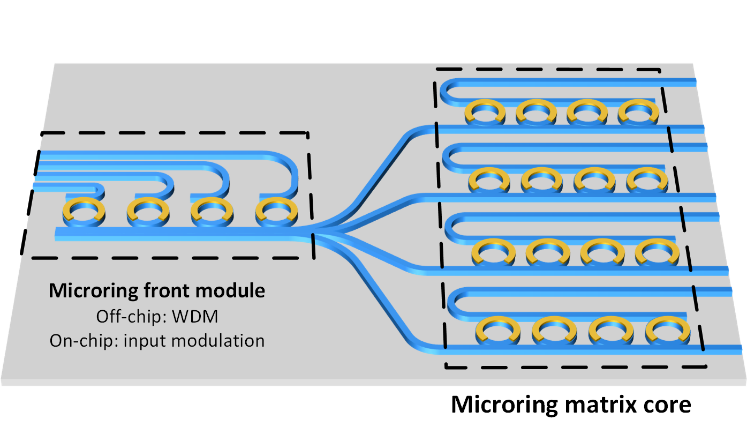
Figure 3. The scheme of WDM-MVM. The microring front module can be positioned off-chip or on-chip, which is designed to serve as a wavelength division multiplexer (off-chip) or to modulate the input vector to different wavelengths (on-chip). The microring matrix core is an N × N-sized microring array corresponding to an N × N-sized matrix.
As one of the basic photonic devices, MZI has been widely used in optical modulators, optical communication, and optical computing. MZI is a natural minimum matrix operation unit, and can be fabricated on a silicon platform to implement the minimum matrix multiplication. We can configure the MZI mesh to simulate the corresponding unitary matrix to realize the optical matrix operation. All row vectors or all column vectors in a standard unitary matrix can form a set of orthonormal basis in the inner product space. A triangular or rectangular MZI mesh can construct a unitary transformation, and then an arbitrary transmission matrix can be constructed through singular value decomposition.
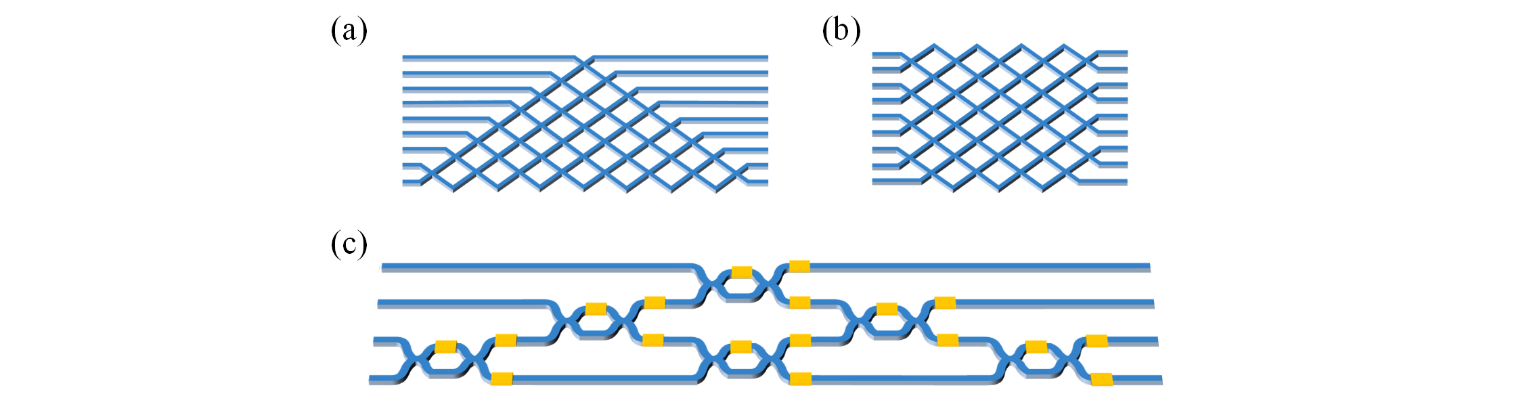
Figure 4. Two decomposition schemes of the MZI matrix core. (a) Triangular and (b) rectangular decomposition schemes. (c) A typical MZI matrix core based on triangular decomposition.
In conclusion, optical computing architectures based on integrated photonic circuits and holography have shown great capabilities for high-speed matrix computing and emerging artificial intelligence applications. However, developing general purpose optical computing systems will remain challenging in the foreseeable future, whose high performance can only be achieved through the flexible design combining hardware with software. On the one hand, chip-scale optical frequency combs, high-speed modulators, and new optical materials can be applied to further improve the performance of the hardware, mainly including computing density, speed and latency. On the other hand, intelligent control algorithms are used to solve the challenges of tunability and practicality. At present, optical computing systems have already been used in computer vision, speech recognition, and complex signal processing, and are expected to expand the frontiers of machine learning and information processing applications.
Nanomaterials recently published a review article on photonic matrix computing written by Professor Xinliang Zhang's group of Huazhong University of Science and Technology as a cover article (Vol. 11, Iss. 7, July 2021). The work was supported by National Natural Science Foundation of China (62075075).
Full text can be viewed by:https://doi.org/10.3390/nano11071683